Managing Business Risks Using Technology
Recently, a team at AXA XL – a global insurance giant that specializes in cargo – was struggling to understand how external influences were adding risk to the process of insuring their customer’s products: specifically, the quality of the container cargo that their clients transported. Cargo loss was a significant source of claims, and with these claims skyrocketing in relation to their earned premiums (known as the ‘loss ratio’), AXA XL needed to find a way to collect and gather any available data that could give them insights into the nature of these losses and how to mitigate the risks that cause them.
AXA XL engaged Parysl, a supply chain data platform, to better analyze the movement of cargo through the entire supply chain. Parsyl began to place sensors inside shipping containers to monitor movement, humidity, and temperature hoping that it would provide information into how disruptions in the supply chain or seasonal changes might impact the quality of the products inside and the potential for risk of loss – and the resulting insurance claims.
The available data and information meant AXA could help clients better anticipate risk and gain more insight into product quality at every point in the supply chain. According to Hélène Stanway of AXA XL, this data-driven approach to risk management led a client to reduce its loss ratio from 180% to 80%.
Digital Transformations are Changing All Aspects of Business
Businesses such as AXA XL are undergoing digital transformations that are fundamentally changing the ways in which risks are mitigated and business value is created. Almost every aspect of running a global business has been transformed in some way by technical solutions to traditional problems. In addition to the emergence of software solutions which impact all operations of a company – from Sales and Marketing, to Customer Support, Engineering, Manufacturing, Accounting and Planning – the rise of Artificial Intelligence has brought a new layer of process automation to bear: increasing operational efficiencies by enhancing human capabilities, and helping to manage the huge volumes of data generated by these new systems.
The COVID-19 pandemic has been an accelerant for these digital transformations. Companies are attempting to upgrade their outdated legacy systems that limit efficiency, create new security issues, and are more costly to maintain. They’re looking to new technologies that provide them cheaper, more effective solutions. Going digital is no longer an option for companies; 87% of senior business leaders believe it’s a top priority moving forward.
Risk Management is Lagging Behind
However, as the adoption of technology to solve business challenges continues to accelerate at a blinding pace, AXA XL seems to have found a relatively untouched aspect of business management: risk identification and mitigation. From financial risk management and supply chain disruptions, to managing competitive threats, product quality, public relations disasters, and environmental and governance missteps, global businesses face many risks that impact their ability to remain profitable and competitive. And on top of this, risk management teams are failing to keep pace with other departments within their organization that are rapidly evolving to adopt new technologies making them more efficient.
The business world is digitizing quickly. The IDC estimates that 175 zettabytes of data will be generated annually by 2025 and businesses are a huge source of this bloat. While this data is generally created with the purpose of creating business value, a heavier reliance on technology and cloud-based data warehouses can also expose companies to more risk as well, including theft of data and other cyber-attacks. As businesses develop their digital capabilities to streamline their processes and improve customer experience, they need to re-imagine how they identify and assess their vulnerabilities.
Challenges in Managing Business Risk Creates a Need
Although legacy systems provide continuity and familiarity within an organization, they are becoming obstacles to the development of data-driven risk solutions. Managers can’t rely on outdated technologies to mitigate modern risks. One of many pressing issues for companies is the threat of data breaches arising from internal and external cyber-attacks, risking the privacy of their customers and the loss of intellectual property and financial information. In 2013, after a data breach at the Royal Bank of Scotland, chief executive Ross McEwan said that “the bank had failed to properly invest in IT”.
And it’s not just the lack of security that is forcing companies to make changes; existing systems — including those that help businesses track and manage risk — are also becoming more expensive to maintain. In a 2018 article written by Jason Miller (@jmillerWFED) for the Federal News Network, he revealed that almost 80% (or roughly $41 billion) of the IT budget for the US government was spent on upgrading and maintaining legacy systems. Although government agencies are now more willing to invest in newer technologies, it doesn’t hide the inefficiencies that arise from using existing IT infrastructure. By continuing to use these old technologies, organizations will lag behind those who invest in the power of AI to deliver more value to customers and keep their business safe. The status quo is not enough.
Krissy Davis, a partner at Deloitte & Touche LLP, argues that businesses that “proactively construct advanced risk management capabilities to keep pace with transformative change have the opportunity to gain significant competitive advantages.” The adoption of these contemporary risk solutions will provide companies a more sophisticated understanding of the numerous risks that can disrupt operations and how to effectively strategize around them.
The Shift to Digital and AI-based Risk Management
A February 2019 report from McKinsey argues that many industries are set to be transformed “using vast amounts of data to build models that improve decision making, tailor services, and improve risk management”. The entire network of connected devices (manufacturing and shipping equipment, sensing and monitoring equipment, location-based devices, tablet, smartphones, access control systems etc.), along with corporate email, messaging, and digital storage systems that are used to share and save information create massive amounts of data that companies can leverage. The combination of these untapped data sources and the growth of computational power over the last decade has created a massive challenge for IT professionals and risk managers. When the available data is understood and business continuity is properly managed, AI will help businesses transform data into valuable risk mitigation strategies, making unexpected risks far easier to anticipate.
Across the world, we are starting to see this shift towards more digital solutions. The United Nations Development Program (UNDP), in collaboration with Indonesia’s National Disaster Mitigation Agency, has started to invest in technologies that are helping to protect people across the country from natural disasters. Through mobile applications and online platforms, they are able to alert people to imminent natural disasters, and even assess the disaster preparedness of schools. Continued investment in these technologies will help governments and humanitarian organizations “address shortfalls in risk management and address challenges before disasters strike.” Although these technologies have much room to grow, the UNDP believes that a digital approach is the way forward for risk management.
The benefits of integrating technology into risk management extend far beyond disaster preparedness, most notably by being quicker and cheaper compared to legacy systems. Automating various day-to-day risk processes prevents risk teams from becoming bogged down in data curation or manual risk identification, and according to Forbes, 51% of executives “admit that cost reduction is the area where AI is delivering first.” The introduction of AI into risk management is a necessary step for managers to realize these savings.
Natural Language Processing Can Help Manage Risk When Legacy Systems Fail
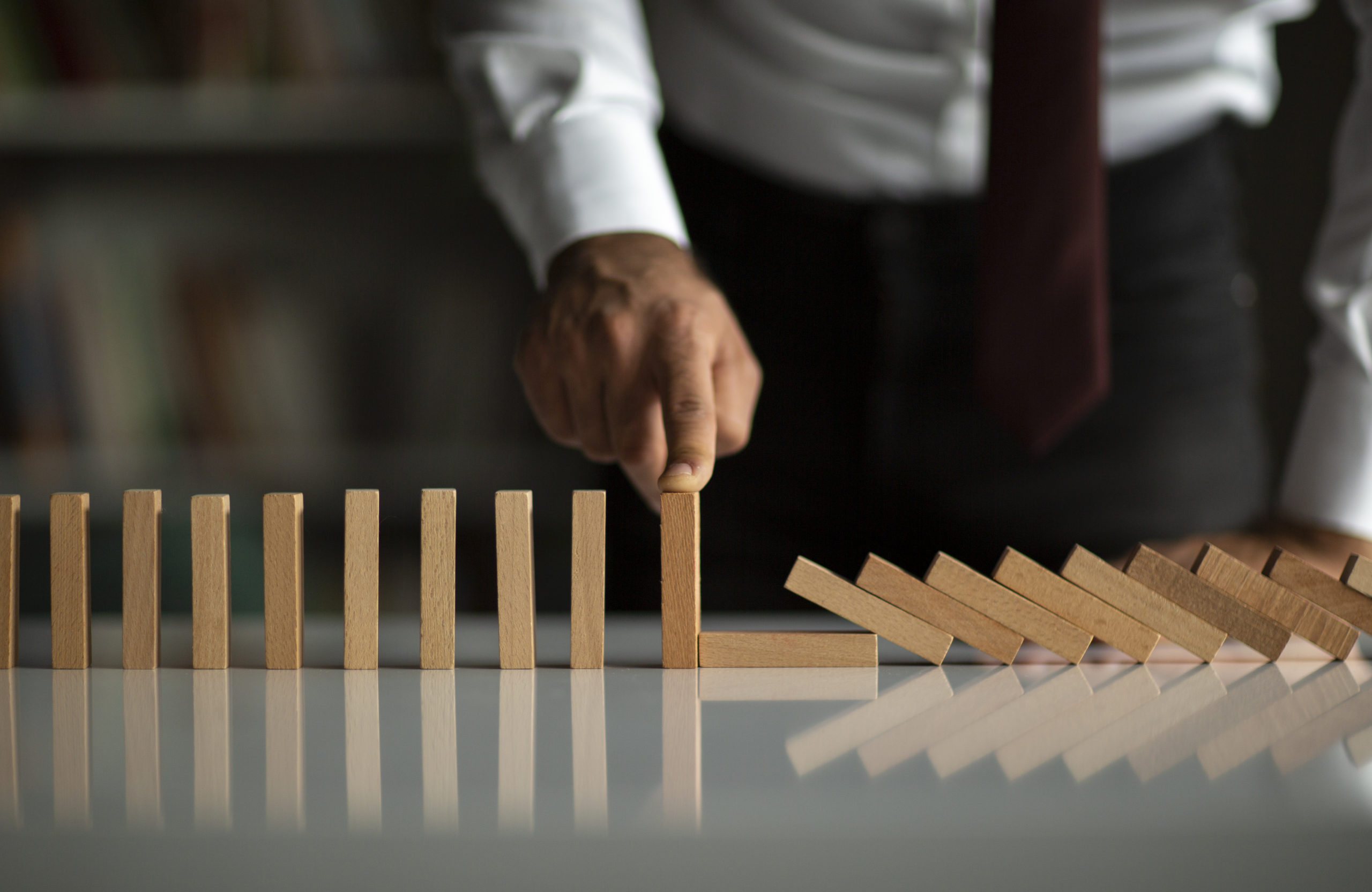
Samir Hans, from the Deloitte Risk & Financial Advisory, believes that “many organizations have done a pretty good job of analyzing and interrogating structured data,” but filtering through unstructured data…is where AI and digital really shine. These unstructured data types can include:
- Online activity related to specific brands that may include negative sentiment – such as social media posts, news articles, press releases, or opinion blogs
- Conversations or mentions that might predict or reveal cyber-attacks on remote areas of the internet
- Online reactions to corporate activities – such as those that might impact a brand’s environmental, social or governance ratings (ESG)
- Information and news from international sources – such as government documents, news articles, or social media activity – can be quickly translated to increase visibility of potential risk events
- Speeches, press briefings, webinars, and other speaking engagements that can be converted into a machine-readable format
Monitoring these unstructured data types through natural language processing “has become a form of risk management and…a useful risk control tool for a variety of businesses”. Having the requisite AI infrastructure to quickly identify and respond to damaging information or negative customer feedback is critical to maintaining a strong brand. According to BrightLocal, a UK-based marketing agency, 92% of buyers are less likely to use a business after reading a negative review, and 40% of customers form an opinion about a brand after reading 1-3 online reviews. A company that has invested years developing a spotless image can see it quickly dissolve if they are not taking a proactive approach to monitoring the unstructured data and sentiment surrounding their brand.
Canadian travel company, Expedia, understands the importance of this firsthand. The company received online backlash when one of its commercials included a violin soundtrack that some social media users called “absolutely obnoxious”. By monitoring reaction to the campaign on social media, news articles, and blogs, Expedia was able to quickly recognize the negative sentiment and shift to a different strategy before the situation could escalate further. (the following ad appropriately featured the same violin being smashed to pieces before being thrown out a window)
In another example, fashion retailer, Ann Taylor, found itself in hot water when social media users complained that while their products “looked good on models, they weren’t so flattering on anyone who wasn’t 5’10 and stick thin.” After immediately identifying the concerns, the company released images of their employees wearing the same products the following day, de-escalating the situation and creating an opportunity to strengthen their image.
Businesses trying to make sense of unpredictable and unstructured data types must invest in NLP capabilities to perform thorough sentiment analysis. Predicting risk is extremely difficult, but with the right infrastructure in place, early detection and response is achievable.
AI and NLP as a Means to Evaluate ESG Reporting
Artificial intelligence and natural language processing also present an opportunity for companies to more effectively monitor their ESG posture. Over the past decade, there has been a steady growth in ESG investing. Executives are warming up to the fact that being “environmentally friendly and socially responsible is good not only for customers and stakeholders, but also for shareholders.”
However, there is a growing concern among business executives – who have little to no control over how their business is evaluated by each rating agency – that each agency “uses their own proprietary methodologies, metrics, weighting, and even definitions of what constitutes ESG.” The system is inherently subjective, and any discrepancies between rating agencies poses problems for companies trying to understand their ESG position. Bank of America, for example, was rated “below average” by one firm, and “well above average by another”.
Artificial intelligence tools can help companies sift through ESG reports, pulling only relevant information to their company, sector, or even geographic location. Performing text classification and sentiment analysis in these reports, repeatedly and at scale, reduces the complexity of a manual review process and presents a more efficient means for companies to determine which insights are most relevant and actionable.
AI For Risk Management: A Double-Edged Sword
As encouraging as AI technologies can be for the future of risk management and ESG monitoring, they are a source of risk as well. A heavy reliance on algorithms trained by biased data sets can lead to flawed decision making and unfair outcomes. Oliver Wyman calls this “garbage in, garbage out” syndrome.
According to Thomas Redman of the Harvard Business Review, “poor data quality is enemy number one to the widespread, profitable use of machine learning.” As more and more data points are entered into machine learning systems, inherent biases become harder to identify and will reinforce biases as the models become smarter. In recent years, the struggle to keep algorithmic biases out of datasets has been magnified as major tech companies such as Facebook, Twitter, Amazon, and Apple have all had to fix, or in some cases, scrap their existing models due to ingrained prejudice.
Redman also emphasizes the fact that biased data can have lasting impacts on the accuracy and credibility of a dataset. Not only does it impact the “historical data used to train the predictive model, [but] in the new data used by that model to make future decisions”. The presence of these unintended biases in machine learning models is an inherent risk that managers must be able to recognize and overcome. If businesses are turning to AI solutions to create more robust decisions or complement their risk and ESG frameworks, it is necessary they understand that the reliability of these decisions could be compromised if the data inputs are biased.
AI Brings Risk Management an Overdue Revolution
It is time for companies to start introducing AI and machine learning into risk management. The amount of information available from the billions of devices in our homes and in our pockets can be used by companies to identify and assess the risks and valuable ESG information that can impact the success of their operations and the safety of their customers. Legacy systems are becoming too archaic and costly to provide these outcomes. In order for businesses to stay competitive, they must integrate AI solutions into their existing ESG and risk frameworks to accelerate their data processing, realize efficiencies, and improve their decision making. While AI provides challenges that can undermine the reliability of outcomes, it should not turn away managers looking to optimize their risk management practices. Whether it be a shipping company trying to reduce its losses, or a school trying to protect itself against natural disasters, AI will be integral to the future of risk management. The quicker companies realize that, the more protected they will be.